Introduction
Reinforcement Learning (RL) has emerged as a powerful technique in the field of artificial intelligence, enabling machines to learn and make decisions through interactions with their environment. Over the years, RL has witnessed significant advancements and has found applications in various domains, ranging from robotics and gaming to finance and healthcare. This blog post explores the latest trends in reinforcement learning, highlighting the innovative applications and advancements that have shaped the field.
2. Reinforcement Learning in Robotics
One of the most exciting applications of reinforcement learning is in robotics. RL algorithms enable robots to learn complex tasks through trial and error, allowing them to adapt and improve their performance over time. This has opened up possibilities for autonomous robots in industries such as manufacturing, healthcare, and logistics.
2.1 Autonomous Navigation
Reinforcement learning has been used to train robots to navigate autonomously in dynamic environments. By learning from their interactions with the environment, robots can develop efficient navigation strategies, avoiding obstacles and reaching their goals effectively.
2.2 Manipulation and Grasping
RL algorithms have also been applied to teach robots how to manipulate objects and perform complex grasping tasks. By learning from trial and error, robots can acquire the necessary skills to handle various objects with different shapes, sizes, and textures.
3. Reinforcement Learning in Healthcare
The healthcare industry has also embraced reinforcement learning to improve patient care and optimize medical treatments.
3.1 Personalized Treatment Plans
Reinforcement learning algorithms can analyze patient data and medical records to develop personalized treatment plans. By considering individual patient characteristics and medical history, RL can optimize treatment decisions, leading to better outcomes and reduced healthcare costs.
3.2 Disease Diagnosis
RL techniques have been used to develop intelligent diagnostic systems that can analyze medical images, patient symptoms, and laboratory results. By learning from a vast amount of data, these systems can assist healthcare professionals in accurate disease diagnosis.
4. Reinforcement Learning in Finance
The finance industry has recognized the potential of reinforcement learning in making better investment decisions and managing risks.
Summary
Reinforcement Learning has gained immense popularity due to its ability to enable machines to learn from experience and improve their decision-making capabilities. This blog post Read Full Article provides an overview of the latest trends in reinforcement learning, showcasing its applications and innovations across different industries.
- Q: What is reinforcement learning?
- A: Reinforcement learning is a type of machine learning where an agent learns to make decisions by interacting with an environment and receiving feedback in the form of rewards or punishments.
- Q: What are the latest trends in reinforcement learning?
- A: Some of the latest trends in reinforcement learning include deep reinforcement learning, multi-agent reinforcement learning, and meta-reinforcement learning.
- Q: What are the applications of reinforcement learning?
- A: Reinforcement learning has various applications such as robotics, game playing, autonomous vehicles, recommendation systems, and resource management.
- Q: What are the innovations in reinforcement learning?
- A: Innovations in reinforcement learning include the use of neural networks for function approximation, improved exploration-exploitation techniques, and the development of new algorithms such as Proximal Policy Optimization (PPO) and Soft Actor-Critic (SAC).
- Q: How does deep reinforcement learning work?
- A: Deep reinforcement learning combines reinforcement learning with deep neural networks to handle high-dimensional state and action spaces. It uses techniques such as deep Q-networks (DQN) and deep deterministic policy gradients (DDPG) to learn complex behaviors.
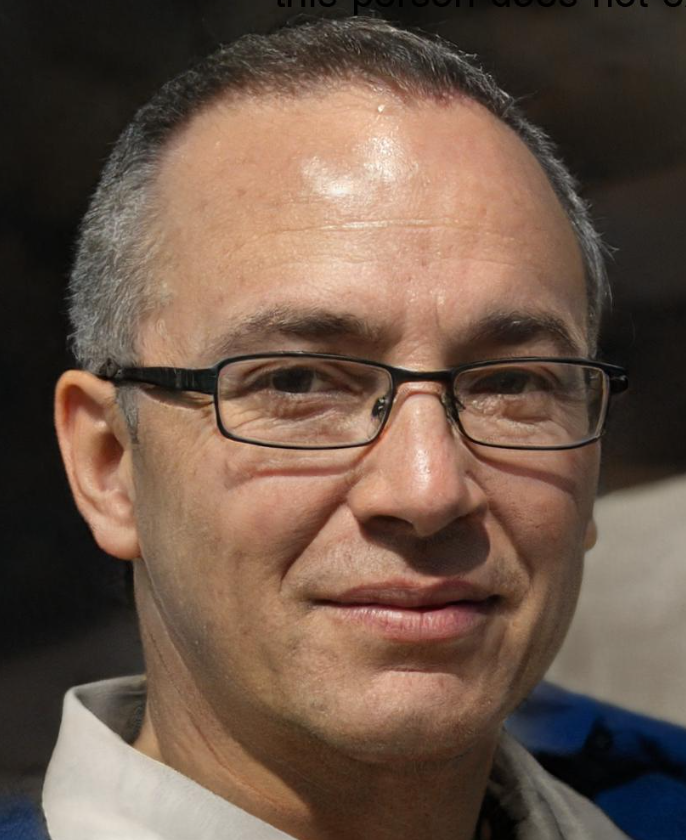
Welcome to my website! My name is Timothy Woodruff, and I am a passionate and experienced IoT Solutions Architect. With a deep understanding of digital transformation, IoT innovations, hardware reviews, and AI updates, I am dedicated to helping businesses harness the power of technology to drive growth and efficiency.